In today’s tech-driven world, artificial intelligence (AI) plays a crucial role in various industries, and our daily lives. However, the story of Mr. M, an Uber Eats delivery driver, highlights the concerns about the unintended biases in AI and the pressing need to address these issues.
The Incident
Mr. M, a delivery driver for Uber Eats, encountered significant challenges due to the company’s AI-powered facial recognition system. As part of his job, he was required to use the app, which occasionally asked him to send selfies to register for jobs. This took a problematic turn after Uber Eats switched to a Microsoft-powered version of their app.
Mr. M began receiving repeated requests to verify his identity. Despite submitting images of himself without any noticeable changes in his appearance, the AI system failed to recognise him. Consequently, Mr. M faced “continued mismatches” in the photos he submitted, leading to his removal from the platform. Unable to access the platform, he was effectively barred from working.
The Allegations
Mr. M believed the AI facial recognition failed to recognise him because he’s black, suggesting that the system discriminated against him. With no opportunity to challenge his suspension, Mr. M initiated legal proceedings against Uber Eats. Before the final hearing, Mr. M settled his claim and was reinstated, allowing him to continue his work.
Broader Implications of AI Bias
Mr. M’s experience underscores a critical issue: AI systems can harbour unintended biases due to the data used for their learning. This type of bias is not confined to facial recognition but can permeate a wide range of AI applications. Biases in AI can result from:
- Training Data: If the data used to train the AI predominantly features certain demographics, the AI may perform poorly on underrepresented groups.
- Algorithm Design: The design of the AI algorithms can unintentionally favour certain outcomes over others.
- Implementation: How AI systems are implemented and the contexts in which they are used can also introduce biases.
These biases can have far-reaching consequences, affecting employment opportunities, access to services, and even interactions with law enforcement.
Recommendations
To mitigate the risks associated with AI biases, organisations should take proactive steps. One crucial measure is to carry out a Data Protection Impact Assessment (DPIA) before rolling out any AI system. A DPIA helps identify and minimise data protection risks. Key steps include:
- Identify the need for a DPIA: Determine if the AI system processes personal data in a way that could result in a high risk to individuals’ rights and freedoms.
- Describe the Information Flow: Outline how data is collected, stored, used, and deleted.
- Identify and Assess Risks: Evaluate potential risks to data subjects and the likelihood of these risks.
- Identify Measures to Address Risks: Implement measures to mitigate identified risks, such as using diverse training data and ensuring transparency in AI operations.
- Document the DPIA Process: Keep detailed records of the DPIA process and decisions made.
By conducting thorough DPIAs and continuously monitoring AI systems, organisations can help ensure their technologies are fair, transparent, and free from discriminatory biases.
Conclusion
The case of Mr. M serves as a reminder of the potential for AI systems to perpetuate biases. Organisations must remain vigilant and take proactive steps, such as conducting DPIAs, to ensure their AI implementations do not inadvertently discriminate individuals or groups. Addressing AI bias is not just a technical challenge but a moral imperative to ensure equity and fairness in an increasingly digital world.
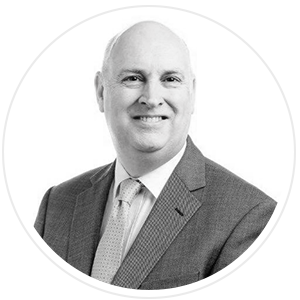
Written by Robert Wassall
Robert Wassall is a solicitor, expert in data protection law and practice and a Data Protection Officer. As Head of Legal Services at NormCyber Robert heads up its Data Protection as a Service (DPaaS) solution and advises organisations across a variety of industries. Robert and his team support them in all matters relating to data protection and its role in fostering trusted, sustainable relationships with their clients, partners and stakeholders.